By Brendan Tierney, Oracle ACE Director @"Brendan"
In a previous article I showed you how you could use the SQL and PL/SQL packages to create and evaluate a data mining model using Oracle Data Mining. This article builds on that previous article and shows you how to use some of the Oracle Data Mining SQL functions. These functions allow us to score or label new data using the Oracle Data Mining model, plus there are functions that allows us to see inside the decision making of the data mining model.
The Oracle Data Miner Data Dictionary Views
A number of Oracle Data Dictionary views exist that allows you to query what Oracle Data Mining models exist in your schemea and the various properties and features of these models. Table 1 lists the data dictionary views for Oracle Data Mining. There are three versions on these data dictionary views (except for the DBA_MINING_MODEL_SETTINGS) and these views allows you to see what ODM objects exist.
|
Dictionary View Name
|
Description
|
|
*_MINING_MODELS
|
This view contains the details of each of the Oracle Data Mining models that have been created. This information will contain the model name, the data mining type (or function, the algorithm used, and some other high level information about the models. This view has the following attributes:
OWNER (for DBA_ and ALL_)
MODEL_NAME
MINING_FUNCTION
ALGORITHM
CREATION_DATE
BUILD_DURATION
MODEL_SIZE
COMMENTS
|
|
*_MINING_MODEL_ATTRIBUTES
|
This view contains the details of the attributes that have been used to create the Oracle Data Mining model. If an attribute is used as a Target this will be indicated by the Target column. This view has the following attributes:
OWNER (for DBA_ and ALL_)
MODEL_NAME
ATTRIBUTE_NAME
ATTRIBUTE_TYPE
DATA_TYPE
DATA_LENGTH
DATA_PRECISION
DATA_SCALE
USAGE_TYPE
TARGET
ATTRIBUTE_SPEC
|
|
*_MINING_MODEL_SETTINGS
|
This view contains the algorithm settings that were used to generate the Oracle Data Mining model for a specific algorithm. This view has the following attributes:
OWNER (for DBA_ and ALL_)
MODEL_NAME
SETTING_NAME
SETTING_VALUE
SETTING_TYPE
|
|
DBA_MINING_MODEL_TABLES
|
This view, which is only accessible by users with the DBA privileges, lists all the tables that contain the meta-data that is related to the data mining models that exist in the database. This view has the following attributes:
OWNER
MODEL_NAME
TABLE_NAME
TABLE_TYPE
|
Table 1 : Oracle Data Mining Data Dictionary Views
Where * can be replaced by
ALL_ contains the Oracle Data Mining information that is accessible to the user.
DBA_ contains the Oracle Data Mining information that is accessible to DBA users.
USER_ contains the Oracle Data Mining information that is accessible to the current user.
Let us now have a look at what these ODM data dictionary views contain for the data mining models that were created in the previous article. The first one being *_MINING_MODELS. This lists the data mining models that you have access to.
SELECT model_name,
algorithm,
build_duration,
model_size
FROM ALL_MINING_MODELS
WHERE mining_function = 'CLASSIFICATION';
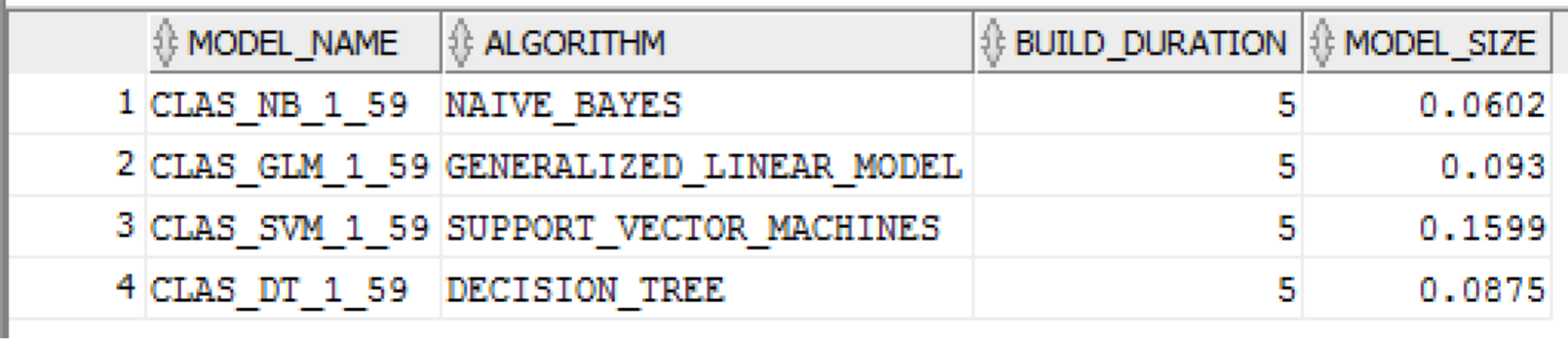
The following example lists what subset of the attributes, from the original data source, are being used by the Oracle Data Mining model. The machine learning algorithms will work out what the most important attribute are from the training data set.
SELECT model_name,
attribute_name,
attribute_type,
data_type,
target
FROM ALL_MINING_MODEL_ATTRIBUTES
WHERE model_name = 'CLAS_DT_1_59';
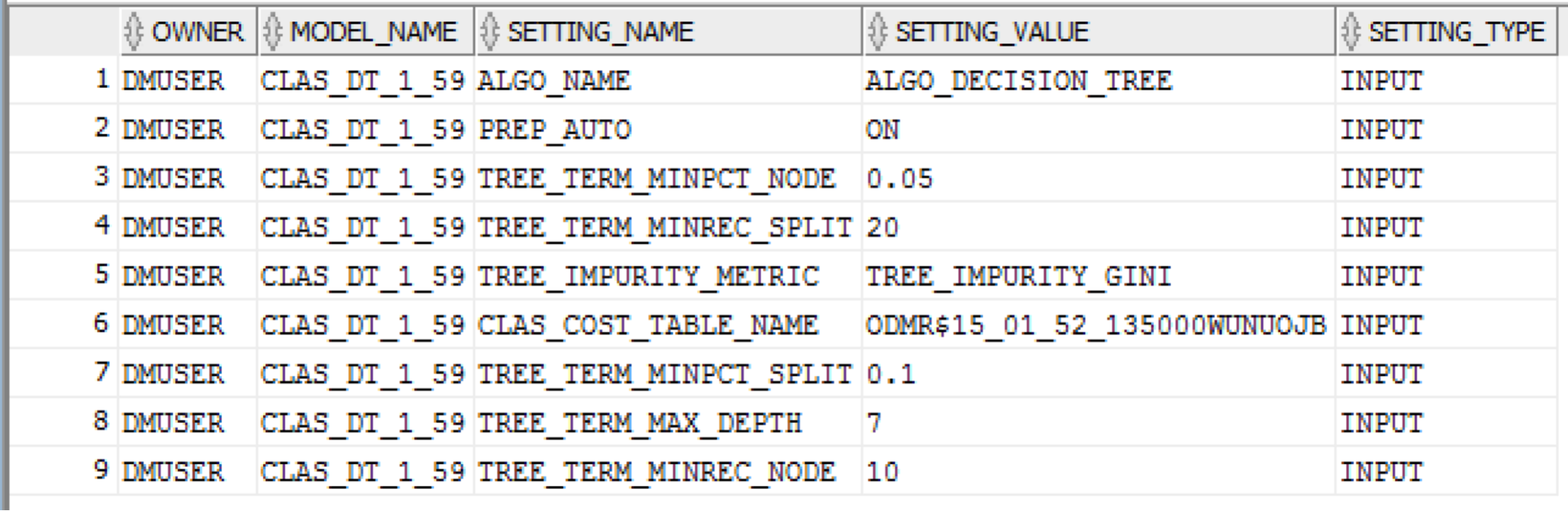
We can also look to see what settings were used for each of our models. This can be useful when you need to rebuild the models. Typically this can happen some weeks/months or a year after the original model was created. The *_MINING_MODEL_SETTINGS view allows us to see what the model setting were so that we can reuse these for the newer versions of the models.
SELECT *
FROM ALL_MINING_MODEL_SETTINGS
WHERE model_name = 'CLAS_DT_1_59';
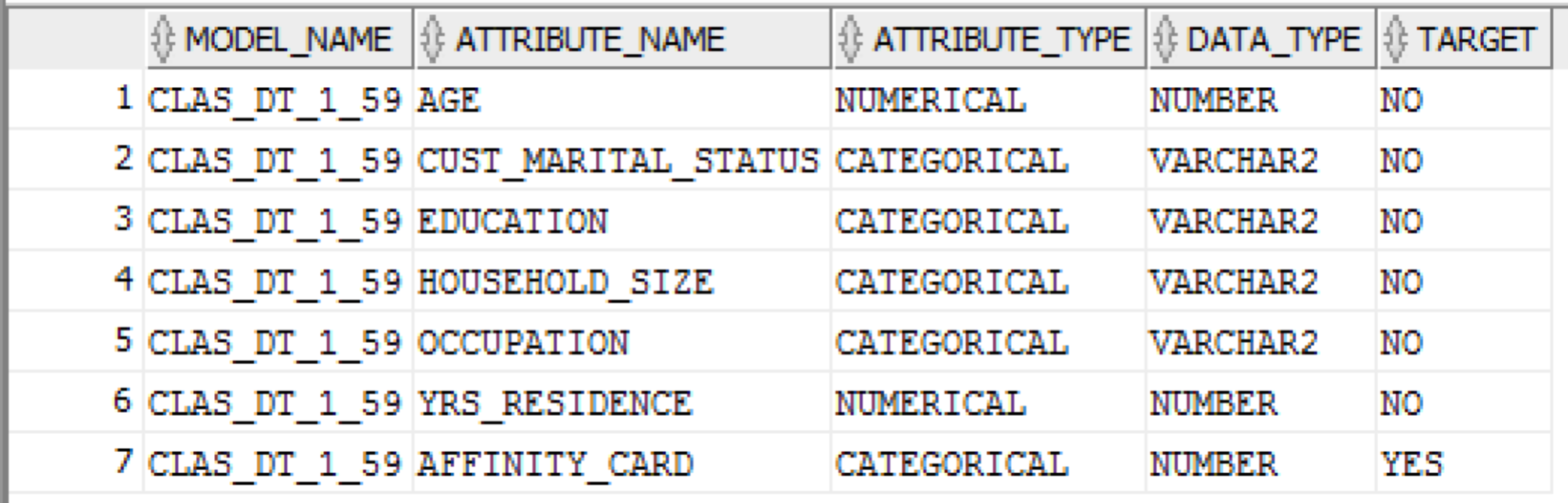
The ODM SQL Functions
SQL functions are available for all the data mining algorithms that support the scoring operation. The functions listed in Table 2 lists all the SQL functions that are available in the Oracle Database to allow you to use your Oracle Data Mining models on new data. These SQL functions come as part of the suite of analytic functions.
|
Function Name
|
Description
|
|
PREDICTION
|
Returns the best prediction for the target.
|
|
PREDICTION_PROBABILITY
|
Returns the probability of the prediction.
|
|
PREDICTION_BOUNDS
|
Returns the upper and lower bounds of the interval wherein the predicted values (linear regression) or probabilities (logistic regression) lie. This function only applies for GLM models.
|
|
PREDICTION_COST
|
Returns a measure of the cost of incorrect predictions.
|
|
PREDICTION_DETAILS
|
Returns detailed information about the prediction.
|
|
PREDICTION_SET
|
Returns the results of a classification model, including the predictions and associated probabilities for each case.
|
|
CLUSTER_ID
|
Returns the ID of the predicted cluster.
|
|
CLUSTER_DETAILS
|
Returns detailed information about the predicted cluster.
|
|
CLUSTER_DISTANCE
|
Returns the distance from the centroid of the predicted cluster.
|
|
CLUSTER_PROBABILITY
|
Returns the probability of a case belonging to a given cluster.
|
|
CLUSTER_SET
|
Returns a list of all possible clusters to which a given case belongs along with the associated probability of inclusion.
|
|
FEATURE_ID
|
Returns the ID of the feature with the highest coefficient value.
|
|
FEATURE_DETAILS
|
Returns detailed information about the predicted feature.
|
|
FEATURE_SET
|
Returns a list of objects containing all possible features along with the associated coefficients.
|
|
FEATURE_VALUE
|
Returns the value of the predicted feature.
|
Table 2 : Oracle Data Mining SQL Functions
In the following sections we will look at some examples of how you can use the three of the most commonly used functions.
Using the PREDICTION SQL Function
The PREDICTION function returns the best prediction for the given model and the data inputted. The syntax of the function is :
PREDICTION ( model_name, USING attribute_list);
The attribute list can be a list of attributes from a table. The ODM model will then process the values in these attributes to make the prediction. This function will process one record at a time to make the prediction and the number of records to process is determined by your query. The following example illustrates how the PREDICTION function can be used to score data that already exists in a table.
SELECT cust_id, PREDICTION(CLAS_DT_1_59 USING *)
FROM mining_data_apply_v
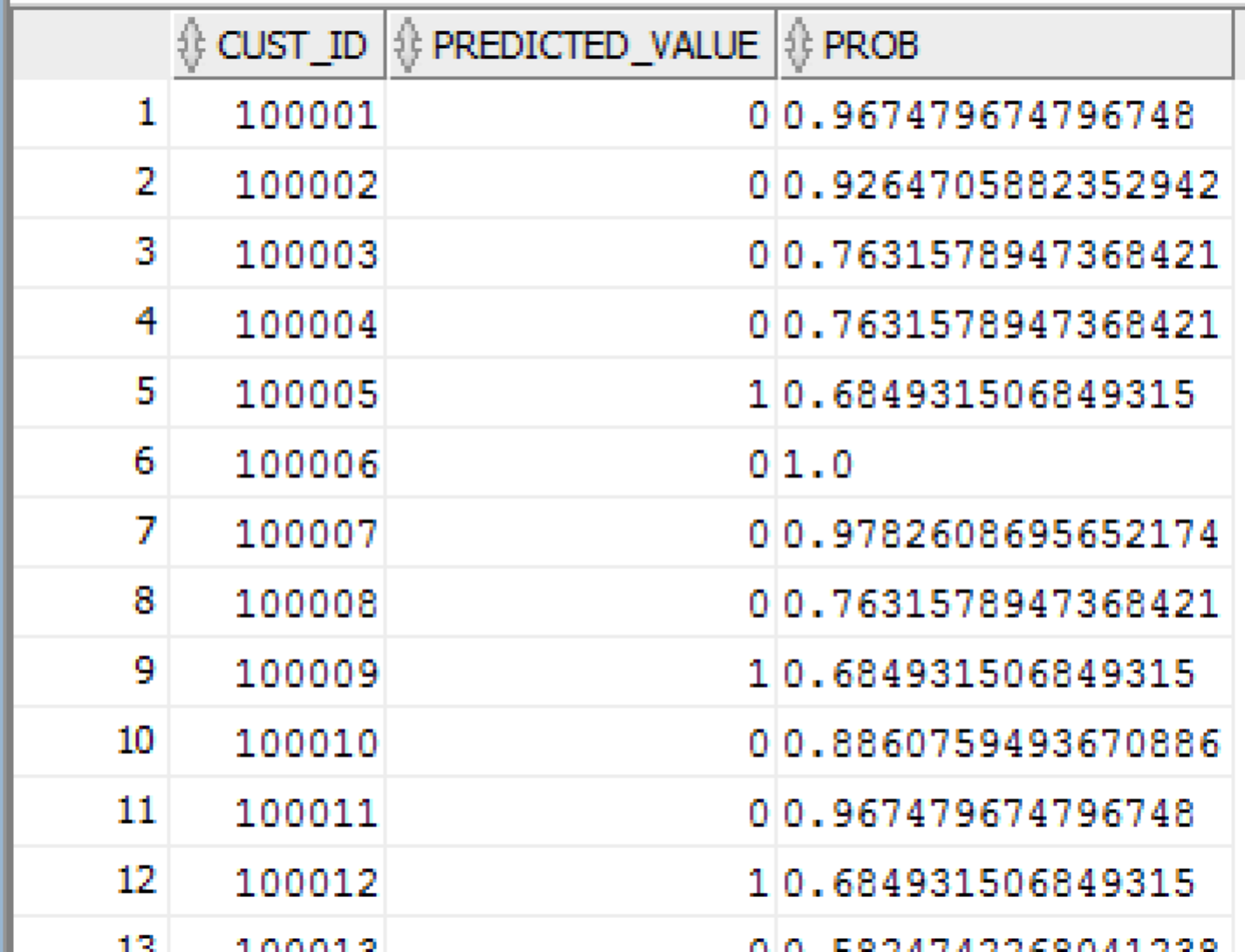
The above example uses the decision tree model we created in the previous section (CLAS_DT_1_59). The USING * takes all the attributes and feeds their values into the decision tree model to make the prediction.
IMPORTANT: Before using these functions you need to ensure that the data being used for scoring will have been prepared in the same way or manner as the data that was used to create the Oracle Data Mining model.
As with all SQL functions you can use them in a variety of ways and in various positions of a SELECT statement. This now allows you to build predictive analytic models into your queries that are involved in your decision making processes.
Using the PREDICTION_PROBABILITIES SQL Function
The second function that we can use is the PREDICTION_PROBABILITY function. This has the same syntax as the PREDICTION function. The syntax of the function is:
PREDICTION_PROBABILITY ( model_name, USING attribute_list);
The PREDICTION_PROBABILTY function will return the value between 0 and 1 and is a measure of how strong a prediction that Oracle Data Mining model thinks it has made. You can use the function in the SELECT and the WHERE parts of a query, just like the PREDICTION function.
SELECT cust_id,
PREDICTION(CLAS_DT_1_59 USING *) Predicted_Value,
PREDICTION_PROBABILITY(CLAS_DT_1_59 USING *) Prob
FROM mining_data_apply_v
ORDER BY cust_id;
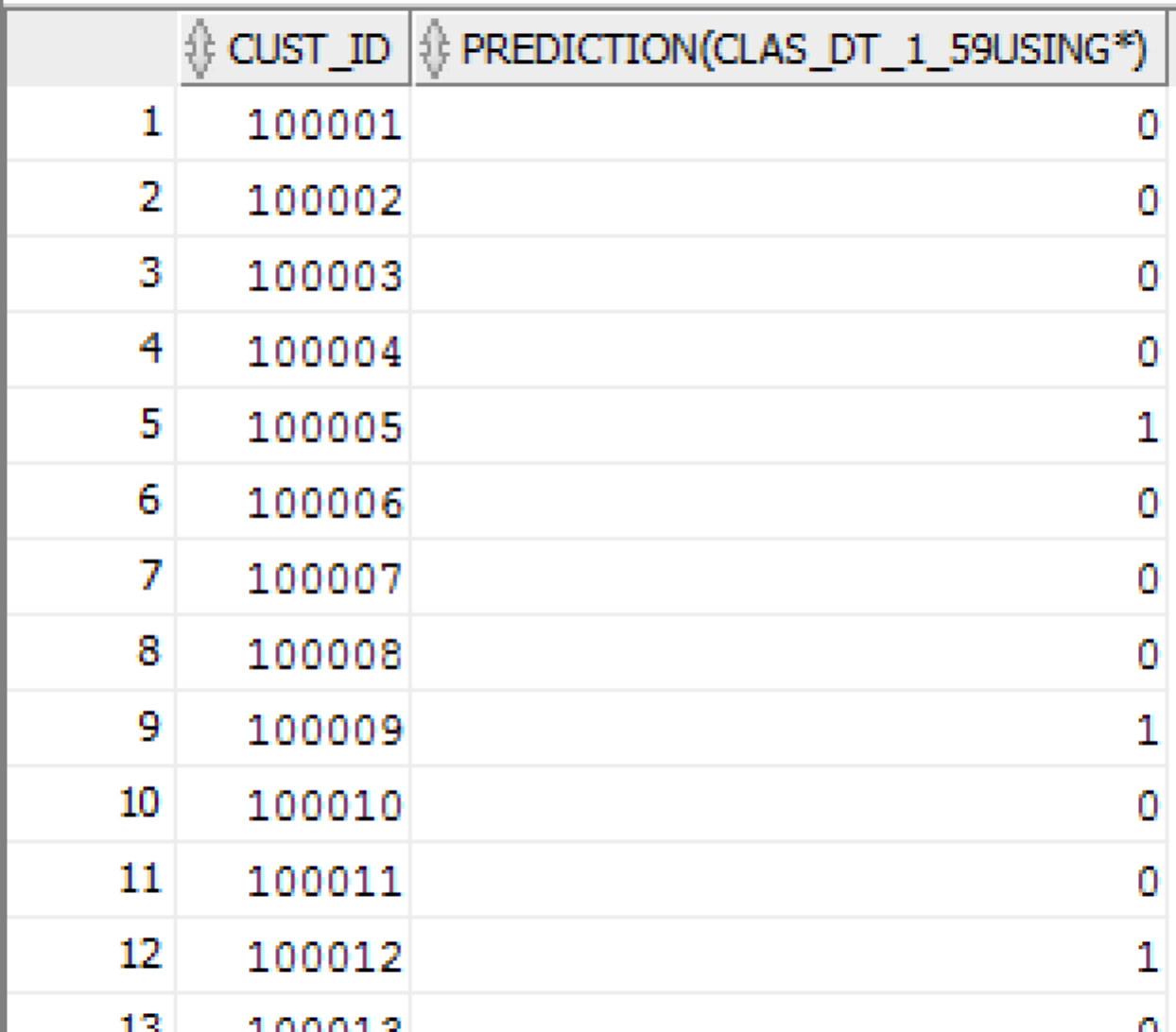
Typically I use this function in the WHERE clause, as it allows me to return an ordered data set in descending probability. This allows me to use this when building workflows and can assign records or customers with different probability scores to different people or process.
Using the PREDICTION_DETAILS SQL Function
What would be useful is to know some of the thinking that the predictive model used to make its thinking. The reasons when one customer may be a "bad customer" might be different to that of another customer. Knowing this kind of information can be very useful to the staff who are dealing with the customers. For those who design the workflows etc can then build more advanced workflows to support the staff when dealing with the customers.
Oracle as a unique feature that allows us to see some of the details that the prediction model used to make the prediction. This functions (based on using the Oracle Advanced Analytics option and Oracle Data Mining to build your predictive model) is called PREDICTION_DETAILS.
When you go to use PREDICTION_DETAILS you need to be careful as it will work differently in the 11.2g and 12c versions of the Oracle Database (Enterprise Editions). In Oracle Database 11.2g the PREDICTION_DETAILS function would only work for Decision Tree models. But in 12c (and above) it has been opened to include details for models created using all the classification algorithms, all the regression algorithms and also for anomaly detection.
The following gives an example of using the PREDICTION_DETAILS function.
SELECT cust_id,
prediction(clas_dt_1_59 using *) pred_value,
prediction_probability(clas_dt_1_59 using *) pred_prob,
prediction_details(clas_dt_1_59 using *) pred_details
FROM mining_data_apply_v
ORDER BY cust_id;
The PREDICTION_DETAILS function produces its output in XML, and this consists of the attributes used and their values that determined why a record had the predicted value. The following gives some examples of the XML produced for some of the records.
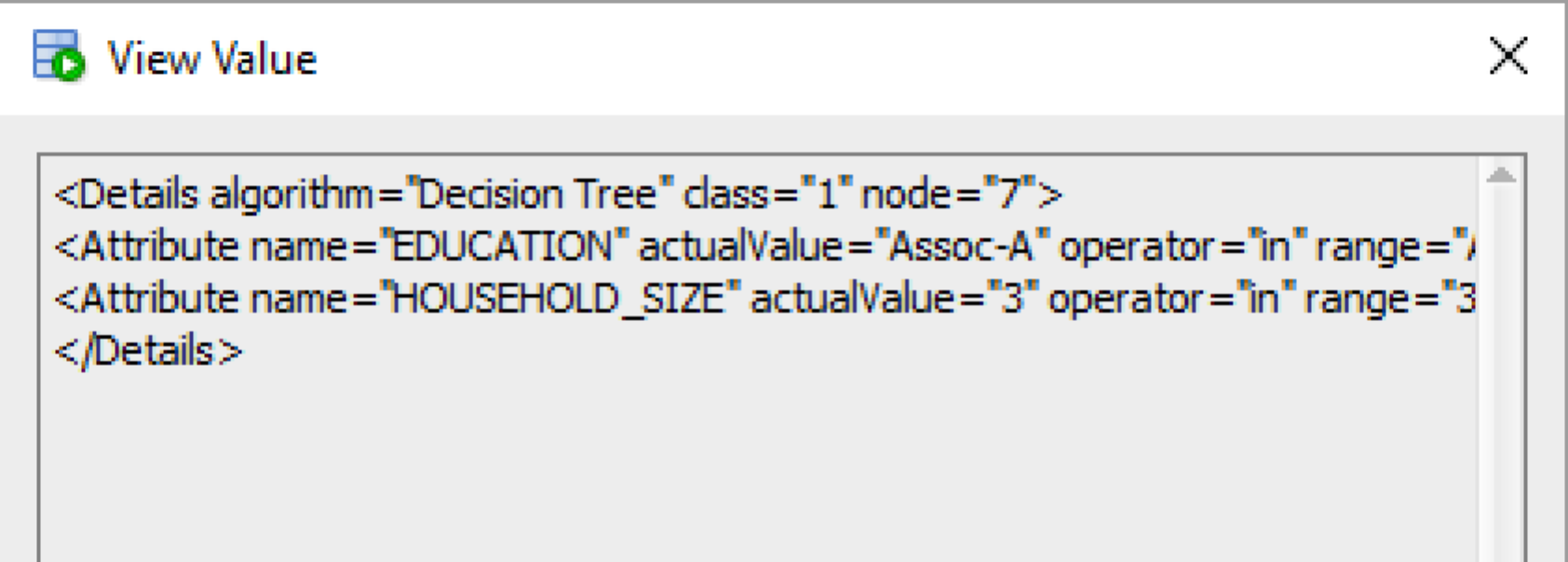
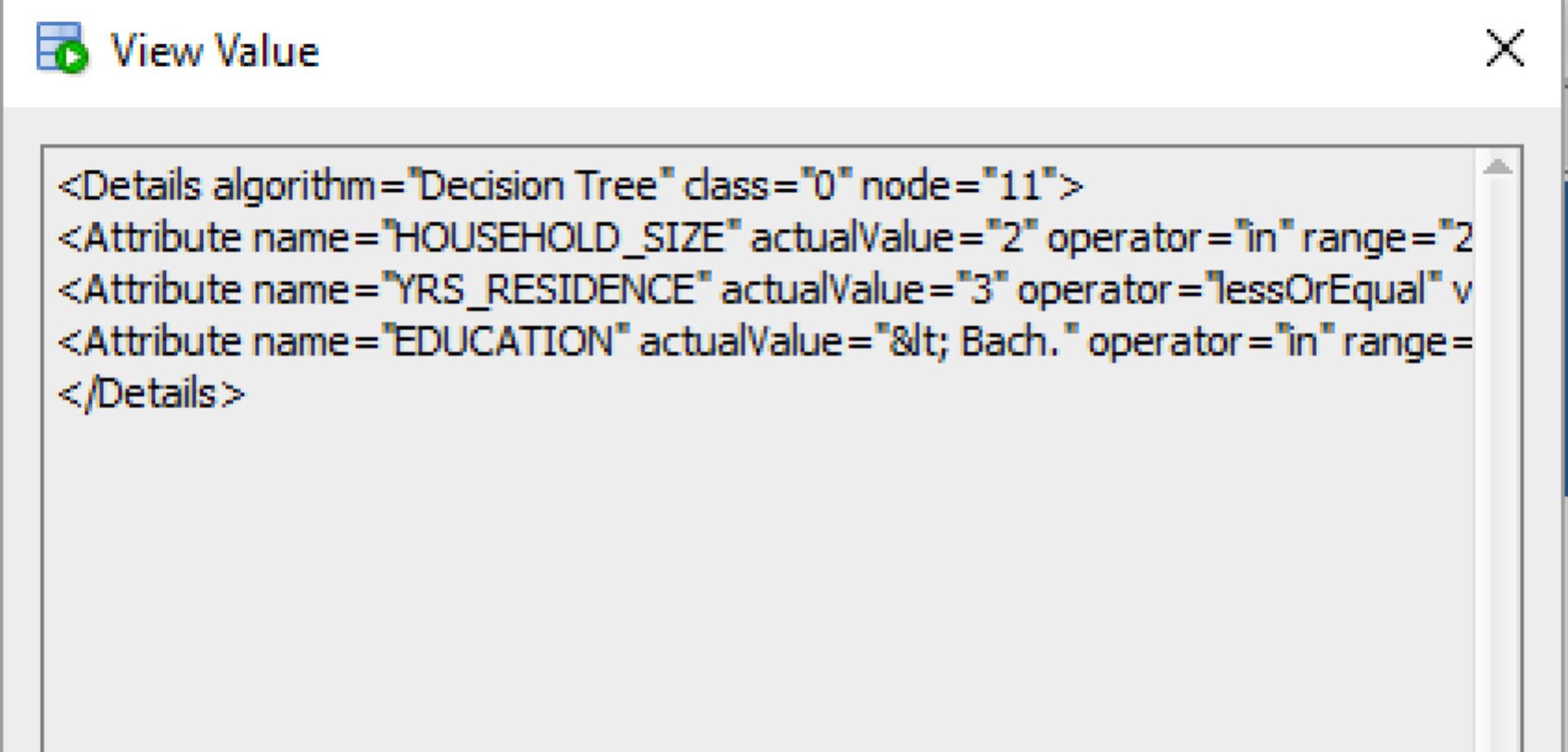
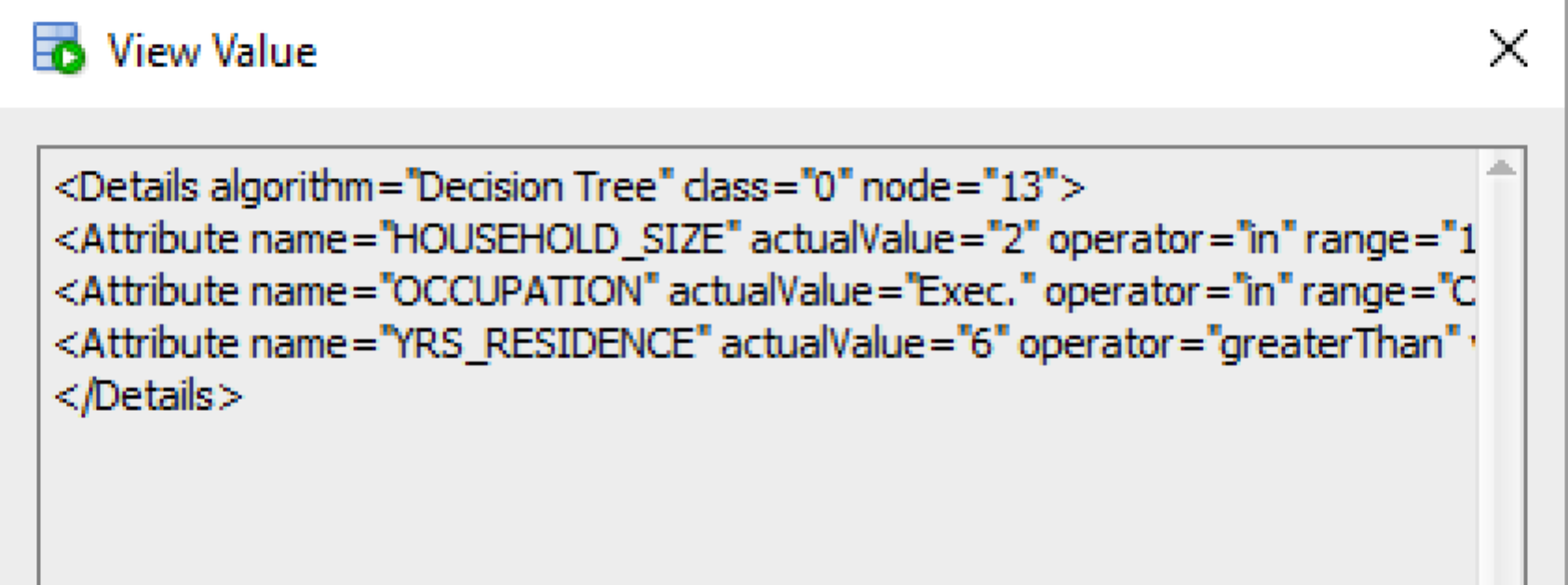
Summary
In this article a number of examples were given that shows you how you can use some of the many SQL functions that allow you to use in-database machine learning models that were created by Oracle Data Miner. The three most commonly used SQL functions where demonstrated in this article. You can follow a similar approach for all the other Oracle Data Mining SQL functions.